By tapping into the power of data analytics coaches can make important decisions more quickly and better prepare athletes for the specific demands of any given race.
By tapping into the power of data analytics coaches can make important decisions more quickly and better prepare athletes for the specific demands of any given race.
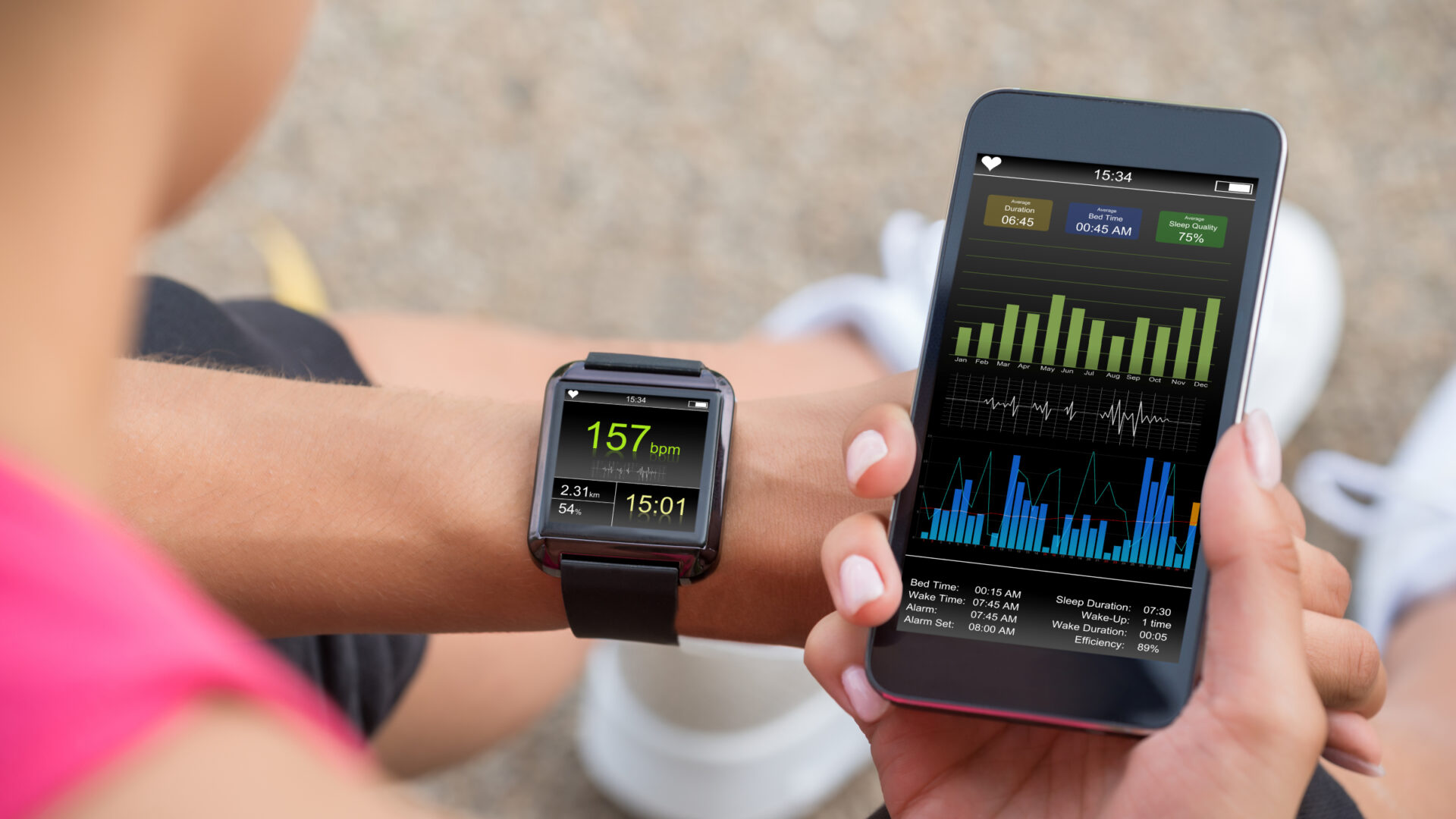